Upcoming Events
CSE Faculty Candidate Seminar - Raphaël Pestourie
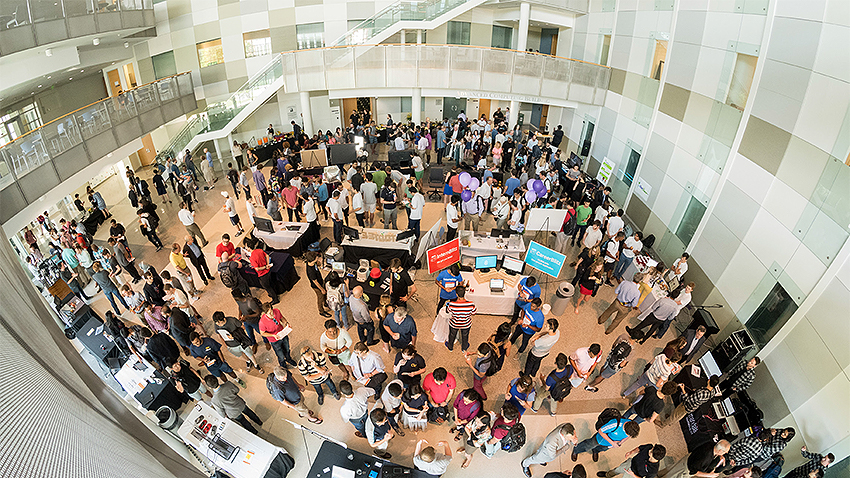
Name: Raphaël Pestourie, Postdoctoral Associate at the Massachusetts Institute of Technology
Date: Thursday, February 23, 2023 at 11:00 am
Location: Coda 230
Link: This seminar is an in-person event only. However, the seminar will be recorded and uploaded to the School of Computational Science and Engineering channel on Georgia Tech MediaSpace following the presentation.
Title: Combining Data and Models for Scientific Machine Learning Methodologies and Inverse Design
Abstract: I present methodologies that combine data and models to accelerate simulations and enable inverse design via scientific machine learning and data-driven approximate solvers. Inverse design is the direct optimization of a target property; it has the potential to automatically discover engineering solutions of real-world problems. However, it is limited by the simulation capabilities of physical phenomena. The centerpiece of these approaches is the creation of data- and resource-efficient global surrogate models that are repeatedly called in the optimization loop.
On the one hand, data-driven models are most often very fast to evaluate, and they do not require a complete knowledge of the process being optimized. Unfortunately, as the number of real-world design parameters increases, these models may require unreasonable amounts of data and resources to be trained accurately. Using scientific machine learning, I show results of an active learning algorithm that reduces the number of training points needed by an order of magnitude to perform inverse design with PDE constraints. By adding information from the physical model via a solver layer in a neural network, one can save an additional order of magnitude in data to perform inverse design. These surrogate models are Physics-Enhanced Deep Surrogates. I also show how scientific machine learning can be leveraged for PDE-constrained inverse design using Physics-Informed Neural Networks and multi-fidelity data.
On the other hand, in many applications, there exist physical models where the simulations are accurate enough to result in a meaningful design, but these simulations may be too resource-intensive to run the optimization process. For example, this is the case for metasurface design–optical devices that present both subwavelength aperiodic patterns and a thousands-of-wavelengths-long diameter. Using a data-driven approximate solver, I show theory and experimental results of large-scale metasurface designs which were enabled by an approximate solver accelerated by a surrogate model; I also show applications of data-driven optics.
Bio: Raphaël Pestourie is a Postdoctoral Associate in the Department of Mathematics at the Massachusetts Institute of Technology. He earned his PhD in Applied Mathematics and an AM in Statistics from Harvard University in 2020. His research interest is inverse design via scientific machine learning and large-scale electromagnetic design, and he was invited to present his scientific machine learning work at multiple upcoming conferences: SIAM Conference on Computational Science and Engineering in Amsterdam, SIAM Conference on Optimization in Seattle, and ECCOMAS-IACM Sicily. Originally from France, Raphaël came to Harvard as an Arthur Sachs Fellow selected by the French Fulbright Commission. While at Harvard, he served as a Resident Affiliate at Quincy House, where he lived among and mentored dozens of undergraduate students.
Event Details
Media Contact
Tasha Thames
tthames6@gatech.edu
EVENTS BY SCHOOL & CENTER
School of Computational Science and Engineering
School of Interactive Computing
School of Cybersecurity and Privacy
Algorithms and Randomness Center (ARC)
Center for 21st Century Universities (C21U)
Center for Deliberate Innovation (CDI)
Center for Experimental Research in Computer Systems (CERCS)
Center for Research into Novel Computing Hierarchies (CRNCH)
Constellations Center for Equity in Computing
Institute for People and Technology (IPAT)
Institute for Robotics and Intelligent Machines (IRIM)